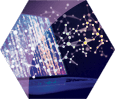
Cambridge Healthtech Institute’s Inaugural
Artificial Intelligence for Early Drug Discovery
How to Best Use AI & Machine Learning for Identifying and Optimizing Compounds and Drug Combinations
April 12, 2019 | Hard Rock Hotel | San Diego, California
This unique one-day symposium on Artificial Intelligence (AI) for Early Drug Discovery will bring together experts from chemistry, target discovery, DMPK and toxicology to talk about the increasing use of computational tools, AI models,
machine learning algorithms and data mining in drug design and lead optimization. The symposium will feature some introductory level talks to bring attendees up-to-speed with how AI is being applied in drug discovery, which will be followed by talks
introducing advanced concepts using relevant case studies and research findings.
Final Agenda
Friday, April 12
7:30 am Registration Open and Morning Coffee
7:55 Welcome and Opening Remarks
Tanuja Koppal, PhD, Conference Director
Marcel Verdonk, PhD, Senior Director, Computational Chemistry & Informatics, Astex Pharmaceuticals
8:00 Integrating Artificial Intelligence and Fragment-Based Drug Discovery
Marcel Verdonk, PhD, Senior Director, Computational Chemistry & Informatics, Astex Pharmaceuticals
We will give a brief overview of Astex’s efforts to integrate AI technology into their fragment-based drug discovery (FBDD) platform. We will also describe our efforts around electrostatic complementarity between protein and ligand, which is critically
important for affinity. We will present AI methodology for generating ultra-fast, near-QM quality molecular electrostatic potential (ESP) surfaces for small molecules, as well as proteins. We will demonstrate the utility of this approach to our FBDD
projects.
8:30 Nature-Inspired de novo Drug Design with AI
Gisbert Schneider, PhD, Professor, Computer-Assisted Drug Design, Department of Chemistry and Applied Biosciences, ETH Zurich
Drug discovery is inspired by natural products. We present automated de novo design for generating novel synthesizable compounds by transfer learning from natural product templates. The chemical synthesis and biological testing positively advocate this
AI concept for prospective application in medicinal chemistry. This presentation will provide first disclosure of prospective natural product-inspired drug design with AI technology.
9:00 Networking Coffee Break
9:30 CASE STUDY: The Power of Networks: Network-Driven Drug Discovery (NDD) and New Chemical Entities
Sree Vadlamudi,
PhD, Business Development & Programme Manager, Discovery, e-Therapeutics plc
We have successfully implemented and validated a highly productive Network-driven Drug Discovery (NDD) approach to identify NCEs in diverse areas of biology. The majority of drug discovery approaches involve the search for a single binding target in a
well-characterised pathway, but that does not reflect the complexity of pathway interactions which occur as a network. We will describe a case study highlighting the application of our proprietary NDD methodology in the discovery and optimisation
of small molecules with a novel mechanism of action.
10:00 CASE STUDY: An Artificial Intelligence Platform for Predicting Voltage Gated Sodium (NaV) Channel Inhibition
David Mowrey, PhD, Senior Research Scientist, in silico Drug Discovery, Icagen
We discuss the development of a machine learning platform for predicting NaV channel inhibition. Here, we explore the use of data augmentation and multitask learning as a means to compensate for the presence of small data sets. We also compare the efficacy
of recurrent neural networks (RNN) on SMILES strings, specifically the long-short term memory (LSTM) classifier compared to more spatially realistic 3D convolutional neural networks.
10:30 CASE STUDY: Combining Systems Biology and AI for Intelligent Drug Design
Aurélien
Rizk, PhD, CTO, InterAx
Our ability to design drugs controlling cellular responses via membrane receptors relies on our understanding of how receptors encode and transfer information. We use mathematical models to combine theoretical knowledge of signaling networks with in-house
generated experimental data. Novel parameters characterizing the mechanistic action of drug molecules can be derived from this cellular systems biology approach. Here, we show how these novel datasets pave the way for new AI applications for drug
design and discovery.
11:00 Deep Learning Applied to Ligand-Based De Novo Design: A Real Life Lead Optimization Case Study
Yann Gaston-Mathé, Co-founder & CEO, Iktos
11:15 Enjoy Lunch on Your Own
12:00 pm Session Break
1:00 Chairperson’s Remarks
Ron Alfa, MD, PhD, Vice President, Discovery & Product, Recursion Pharmaceuticals
1:05 Re-Imagining Drug Discovery through AI
Ron Alfa, MD, PhD, Vice President,
Discovery & Product, Recursion Pharmaceuticals
Massively expanding and accelerating traditional approaches like phenotypic screening provide a feasible near-term solution to bringing substantial improvements to the efficiency of discovery and development efforts. This talk will detail how Recursion
sees the use of AI in drug discovery and will describe some technical strategies to accelerate discovery using AI, including our image-based phenotypic screening platform. The use of deep learning models to build predictive tools for multiple stages
in the drug discovery pipeline will be discussed.
1:35 Design of an Artificial Intelligence System for Drug Discovery
Istvan Enyedy, PhD, Principal Scientist, Biogen
Artificial intelligence systems have the potential of accelerating drug discovery by increasing the time scientists spend on designing the candidate for development. Multiple machine learning models can be used for driving multiparameter optimization.
The use of statistical analysis of the machine learning models in an AI system provides information about the reliability of the predictions and helps in the decision-making process.
2:05 Recognizing the Promise of Adaptive Intelligence Using Mechanistic Modeling
Jo Varshney, DVM, PhD, Founder, CEO, VeriSIM Life
We’re already seeing the impact of Artificial Intelligence adoption within healthcare, however the true potential of personalized medicine is constrained by the complexities of human/animal physiology. VeriSIM Life is complementing machine learning
algorithms with the knowledge of biological systems to address this rate limiting step for drug development. The integration of differences in demographics, genetics, disease progression, and co-medication is enabling us to make critical decisions
earlier than ever in the drug development process.
2:35 Networking Refreshment Break
3:05 FEATURED PRESENTATION: A Case Study in Machine Learning: Integrating Metabolism, Toxicity, and Real-World Evidence
S. Joshua Swamidass, MD, PhD, Associate Professor, Department of Immunology and Pathology, Division of Laboratory and Genomic Medicine; Faculty Lead, Translational
Informatics, Institute for Informatics, Washington University
Many medicines become toxic only after bioactivation by metabolizing enzymes, sometimes into chemically reactive species. Idiosyncratic reactions are the most difficult to predict, and often depend on bioactivation. Recent advances in deep learning can
model bioactivation pathways with increasing accuracy, and these approaches are giving us deeper understanding of why some drugs become toxic and others do not. At the same time, deep learning can be used to understand drug toxicity as it arises in
clinical data and why some patients are affected, but not others.
3:35 Modeling in Drug Metabolism for Drug Design and Development
Hao Sun,
PhD, Principal Pharmacokineticist, DMPK, Seattle Genetics
Several categories of modeling approaches have been applied to drug metabolism. The talk will focus on: 1. structure-based molecular modeling with crystal structures of drug metabolizing enzymes for drug design and lead optimization; 2. data mining of
high-resolution mass spectrometric data for metabolite identification; 3. pharmacokinetic modeling for preclinical in vivo study design; and 4. PK/PD modeling for dose prediction. These modeling approaches have significantly improved efficiency in
drug metabolism-focused drug discovery and development.
4:05 Quantitative Prediction of Complex Drug-Drug Interactions Involving CYP3A and P-glycoprotein: A Case Study of Anticancer Drug Bosutinib
Shinji Yamazaki, PhD, Department of Pharmacokinetics, Dynamics and Metabolism, La Jolla Laboratories, Pfizer Worldwide Research and Development
Physiologically-based pharmacokinetic (PBPK) modeling is a powerful tool to quantitatively predict DDIs based on drug-dependent physicochemical and pharmacokinetic parameters with drug-independent physiological parameters. There is growing emphasis in
developing PBPK models to assess potential risks on DDIs of new molecular entities. This presentation highlights a quantitative PBPK modeling approach to understand complex DDIs of bosutinib via not only CYP3A-mediated metabolism but also P-glycoprotein-mediated
efflux on absorption.
4:35 Close of Conference